Managing hospital staffing is a complex and dynamic challenge. Nursing leaders put in significant effort to ensure the right number of nurses are available for each shift, continuously adjusting to changes in patient admissions, discharges, and clinical needs. However, the chaotic nature and constant variability in any hospital environment makes it difficult to predict patient volume and staffing demands with absolute precision.
The Challenge: Manual Scheduling and Its Limitations
Nursing leaders rely on experience, real-time hospital conditions, and historical trends to make staffing decisions. However, the number of patients fluctuates due to:
✅ Unexpected admissions and discharges – Emergency cases, early discharges, and changes in patient conditions.
✅ Surgical and procedural schedules – The complexity of cases and required recovery times vary significantly.
✅ Daily and seasonal trends – Different patterns emerge on weekends, holidays, and during flu season.
🔹 Example: Maria, an experienced nurse manager, spent hours adjusting shift schedules manually, only for patient volumes to change at the last minute. Despite her professionalism, last-minute changes made scheduling unpredictable. This led to 'shifts-on-target' reaching just 57%, far from ideal.
How AI Predicts Census and Workload
To make staffing proactive rather than reactive, In-House Health’s AI model predicts the number of patients per shift and their expected workload. The system processes a combination of historical data and real-time factors, including:
📌 ADT (Admissions, Discharges, Transfers) Data – Where did a patient stay?
📌 Perioperative Data – What procedure was performed, and which surgeon conducted it?
📌 Time & Shift Variables – Morning, day, night shifts, weekdays vs. weekends.
📌 Patient Demographics – Age, diagnosis, and other key characteristics.
📌 Historical Trends – Previous census patterns to anticipate future needs.
Predicting Length of Stay (LOS)
To estimate the likelihood of a patient staying in the hospital after a certain number of hours, we analyze historical data to determine the average length of stay for specific procedures and surgeons (among multiple other factors).
🔹 Example: A patient who undergoes a Craniotomy performed by Doctor X is likely to stay in the hospital for 150 hours.
This allows us to create a probability distribution of how long a patient will stay:
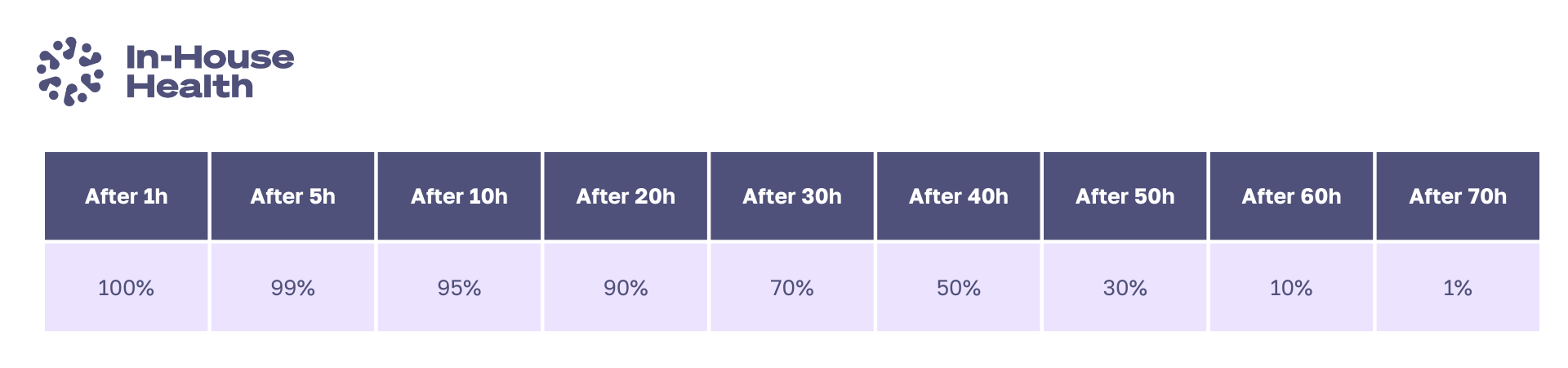
Department Matcher Model
Once we estimate how long a patient will stay, we predict where they will be positioned within the hospital.
We assign probabilities to different departments based on:
- ADT Data – Where did a patient stay?
- Perioperative Data – What procedure was performed, and which surgeon conducted it?
🔹 Example: A patient undergoing Сatheterisation/Ablation has a 90% chance of being placed in the Heart-Chest department and 10% in the Daily-Tzintur department.
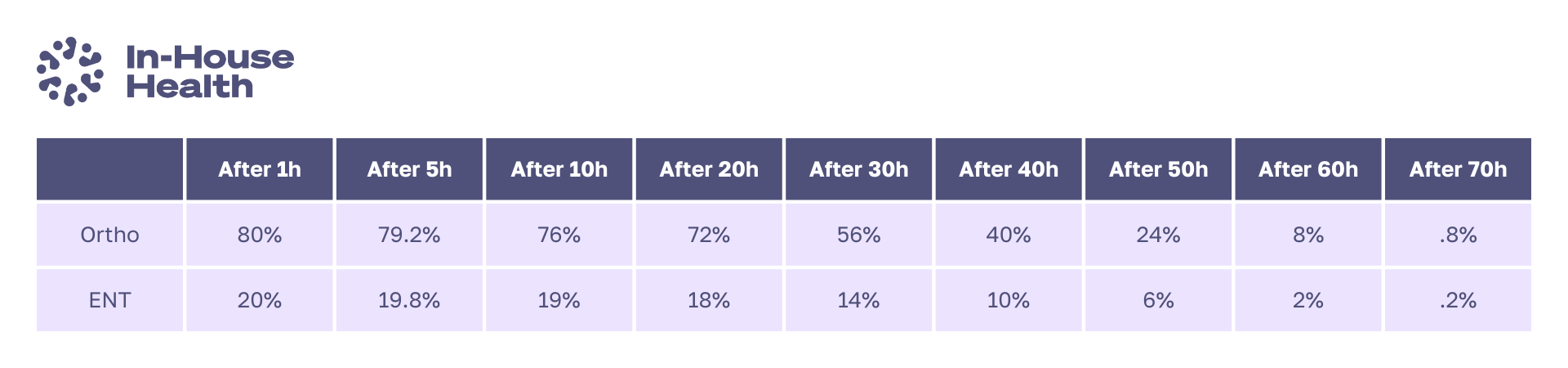
Aggregating Predictions for Staffing Decisions
By applying this model to every scheduled patient, we create a big table of expected patients per department per hour. This generates a forecasted patient census per department and shift, giving nursing leaders a clearer picture of anticipated workloads. Maria, the nurse manager, now had data-backed insights at her fingertips. Instead of adjusting schedules manually, she could anticipate patient volume shifts week in advance.
Day Shift Corrective Algorithm
To improve accuracy, AI continuously refines forecasts based on:
- Current prediction values
- Day of the week
- Shift type (morning/afternoon/night)
- How many days ahead the forecast is being made
This dynamic learning ensures that schedules remain adaptable and precise as new data comes in.
Results: AI-Driven Scheduling in Action
Using In-House Health’s predictive AI model, the same unit saw major improvements:
✔ Optimally staffed shifts increased from 57% to 70%.
✔ Total shifts required decreased by up to 10%.
✔ Nurse managers finalized accurate schedules much earlier.
✔ Improved nurse satisfaction and reduced last-minute schedule changes.
Why This Matters
💡 Supporting nursing leaders: AI provides real-time data insights to help optimize staffing decisions.
💡 Predicting fluctuations: Instead of reacting to census changes, hospitals can anticipate them and prepare accordingly.
💡 Reducing inefficiencies: By consolidating complex data, the system reduces manual adjustments and last-minute scheduling changes.
Conclusion: The Future of Nurse Scheduling is Predictive
Hospitals face ever-changing patient loads, making predictive staffing essential. AI-driven models provide a structured way to analyze, learn, and adapt to fluctuations, ensuring nursing teams have the right support at the right time.
Maria no longer spent hours manually adjusting schedules — AI gave her the power to make informed, proactive staffing decisions with confidence.